The objective of the proposal is to develop a hybrid laser and vision-based NDI system (HLV-NDI) for damage inspection and quantification. The innovations will overcome current major obstacles for rapid large area detection and characterization of either manufacturing or in-service damage in composite structures in terms of sensitivity, resolution, and accuracy.
A hybrid laser and vision-based NDI system (HLV-NDI)
A hybrid laser and vision-based NDI system (HLV-NDI) including following sub-systems: 1. laser-based scanning interferometry system (SLDV) and 2. vision-based ultrasonic interferometry system (Boost ESPI/Ultrasonic shearography).
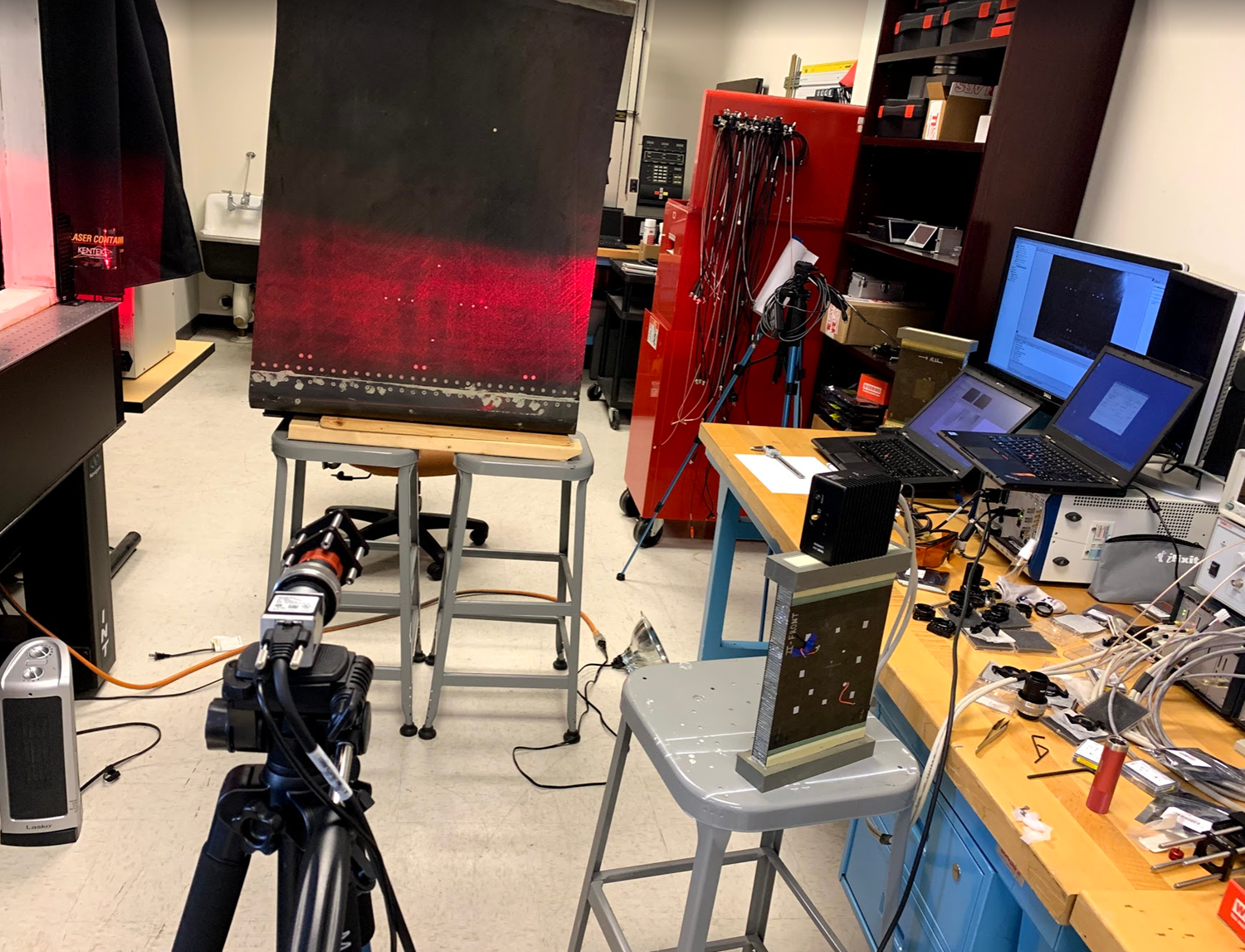
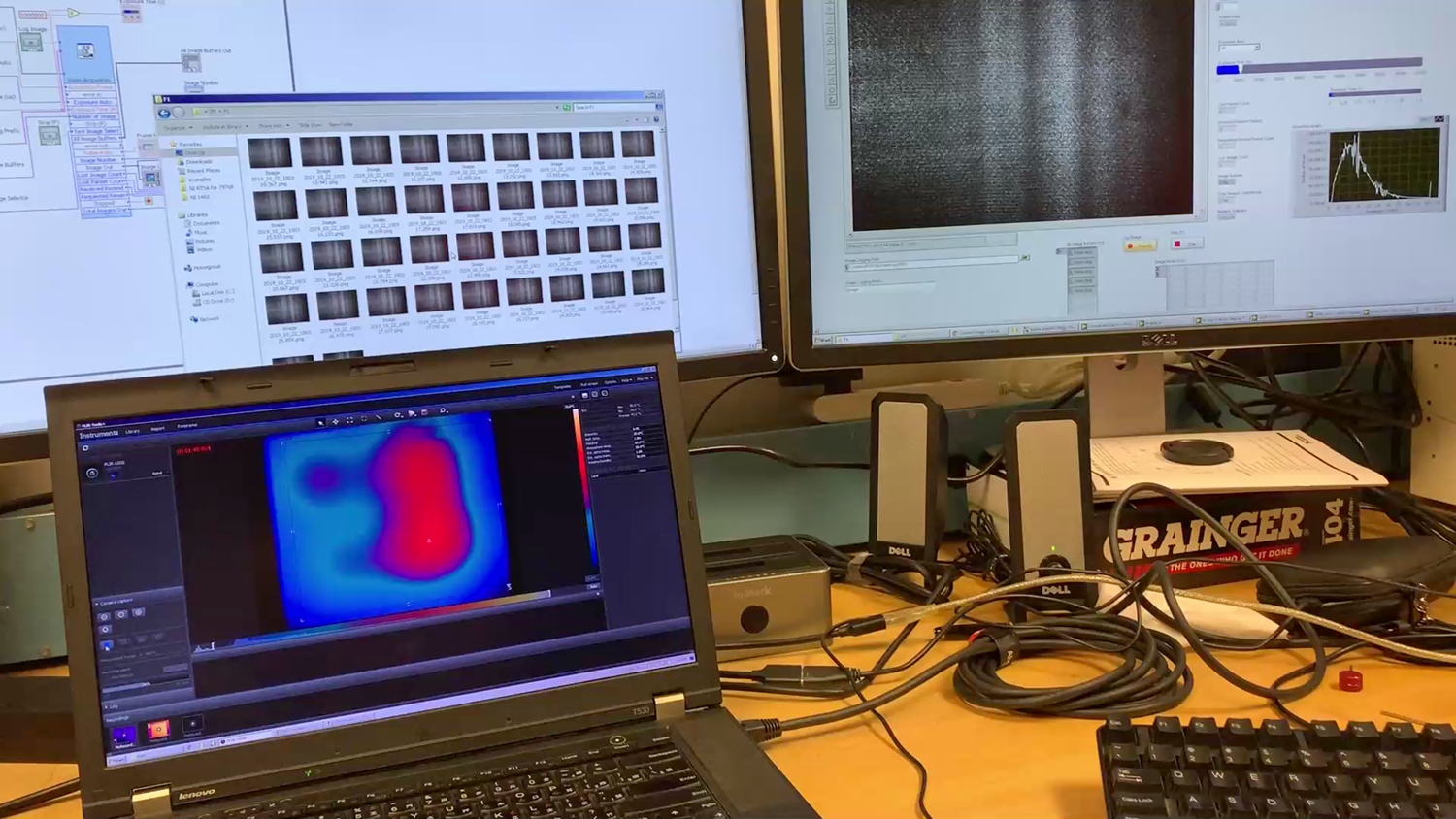
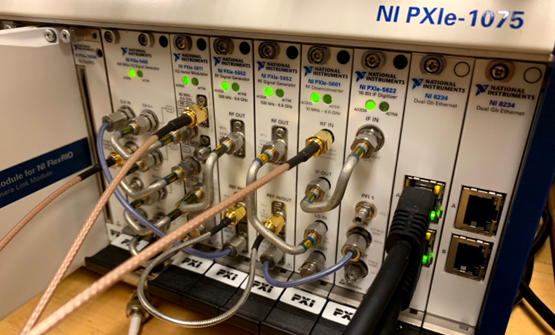
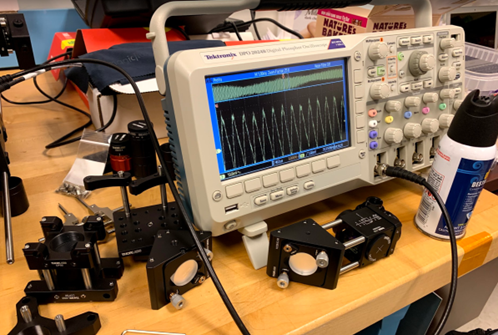
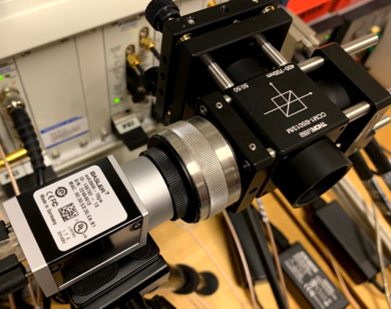
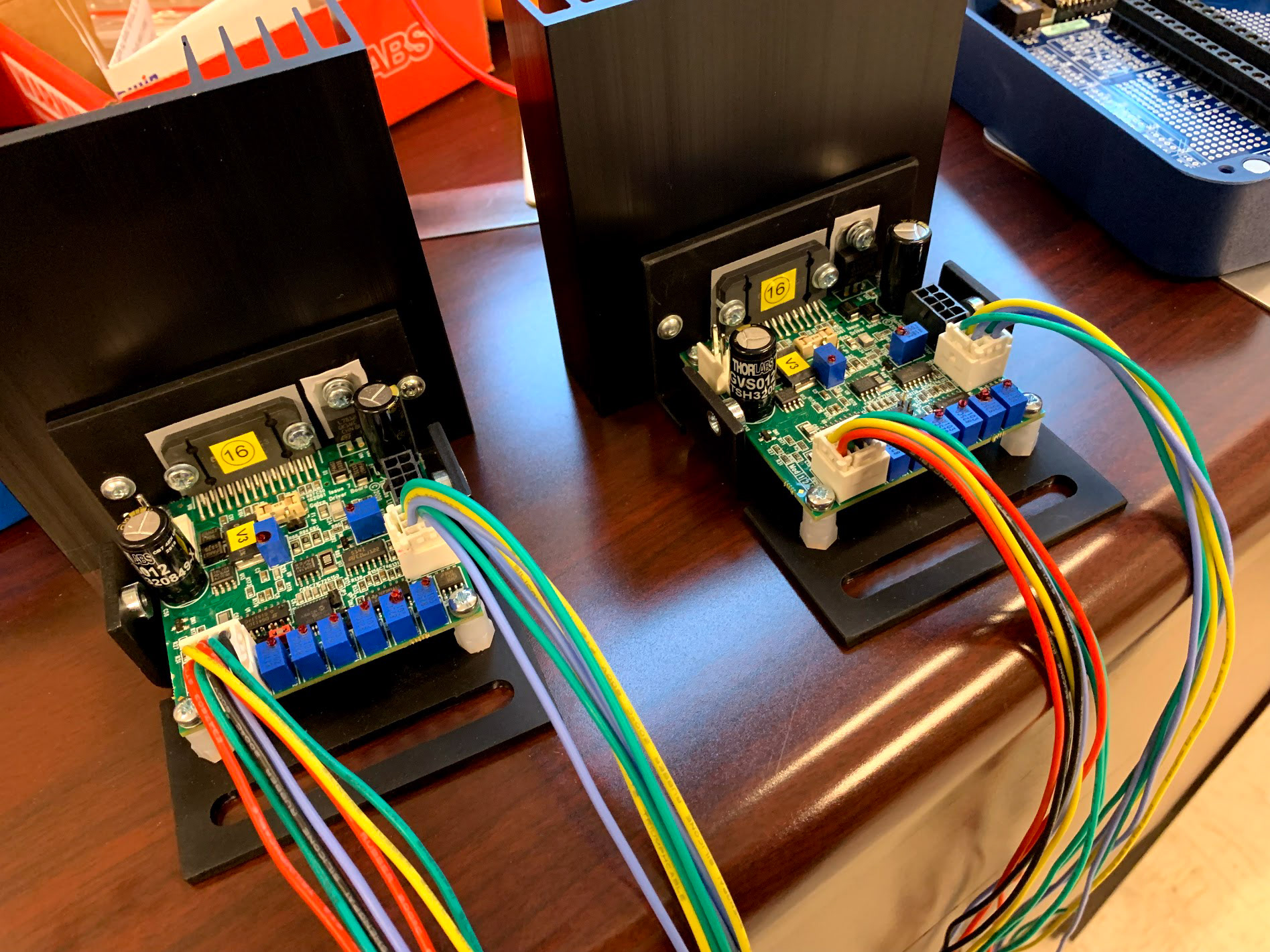
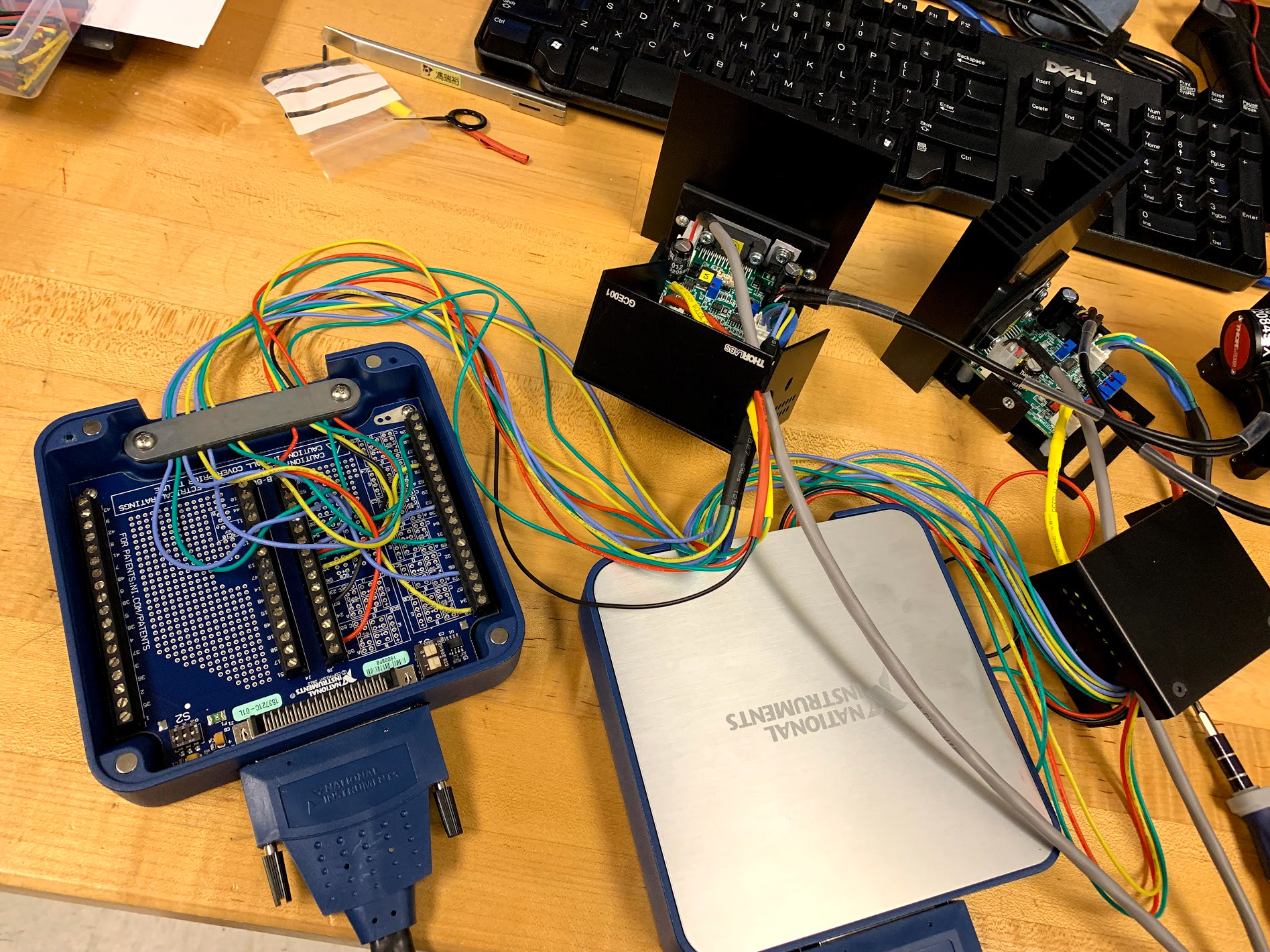
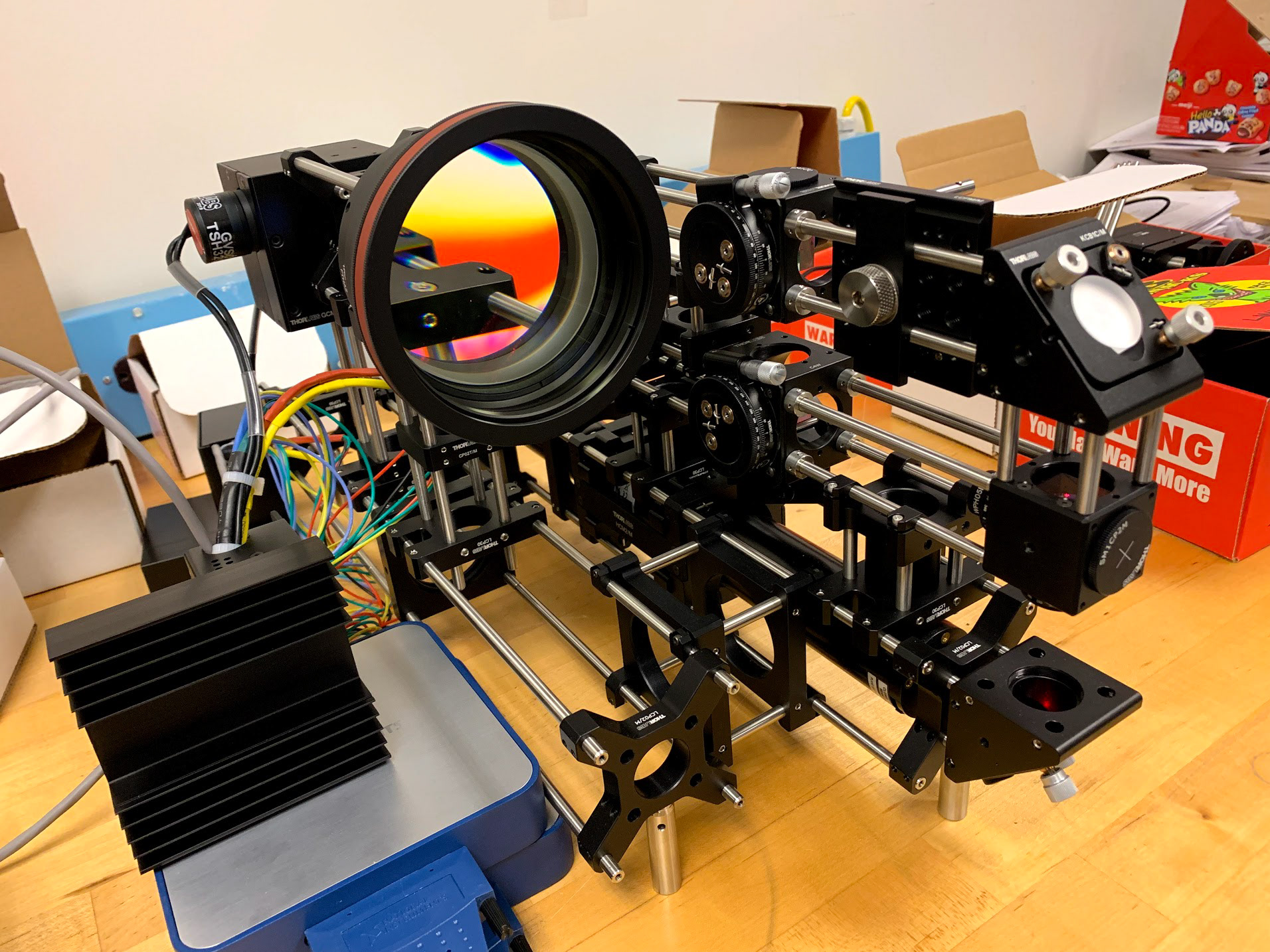
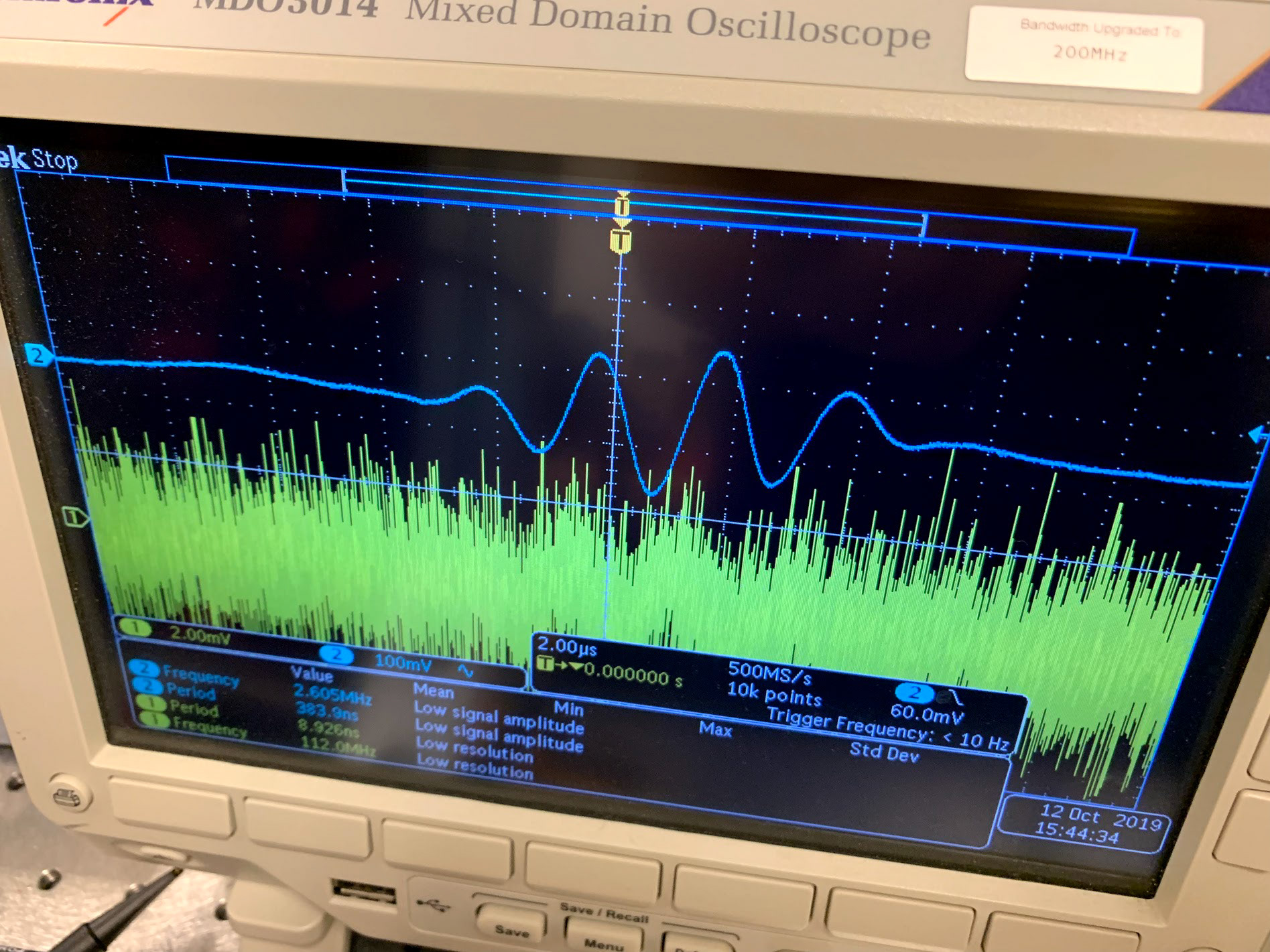
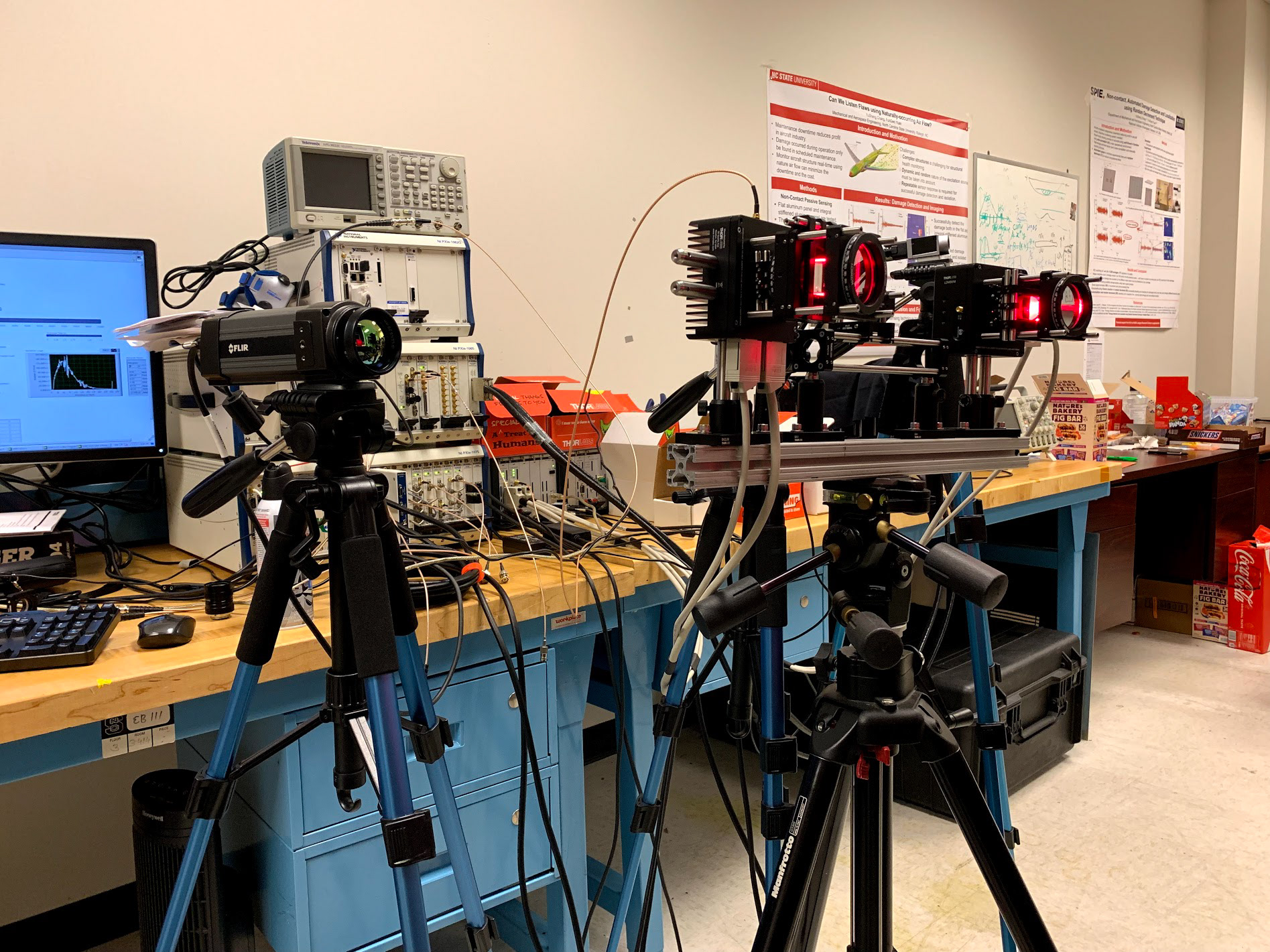
Electronic Speckle Pattern Interferometry (ESPI)
Electronic speckle pattern interferometry (ESPI) also known as TV Holography, is a technique which uses laser light, together with video detection, recording and processing to visualise static and dynamic displacements of components with optically rough surfaces. The visualisation is in the form of fringes on the image where each fringe normally represents a displacement of half a wavelength of the light used (i.e. quarter of a micrometre or so).
ESPI can be used for stress and strain measurement, vibration mode analysis and nondestructive testing. ESPI is similar to holographic interferometry in many ways, but there are also significant differences between the two techniques.
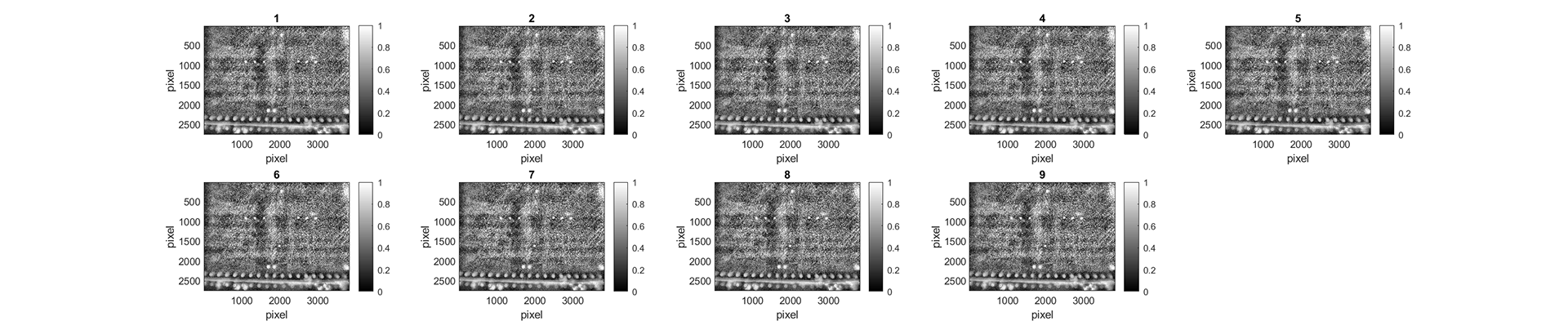
Speckle imaging in time series
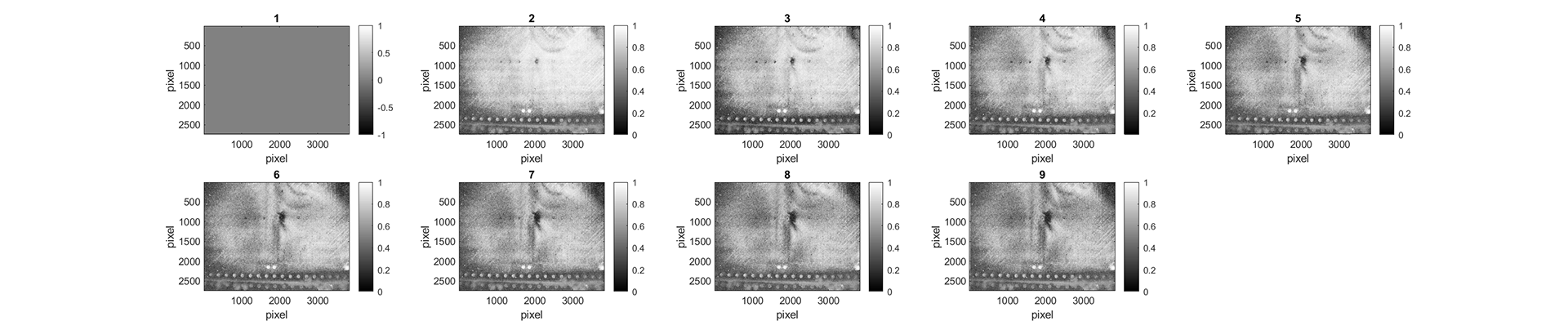
Riesz phase correlation in time series
Multi-sensor Data Fusion
The core of HLV-NDI is based on multi-sensor data fusion. Multi-sensor data fusion is also known as sensor fusion (a subset of information fusion) which combines sensory data or data derived from disparate sources such that the resulting information has less uncertainty than would be possible when these sources were used individually. Multi-sensor data fusion techniques not only integrates the hardware but also at signal processing stage to enhance system performance throughput and overcome traditional modalities of data representing in time and space for imaging.
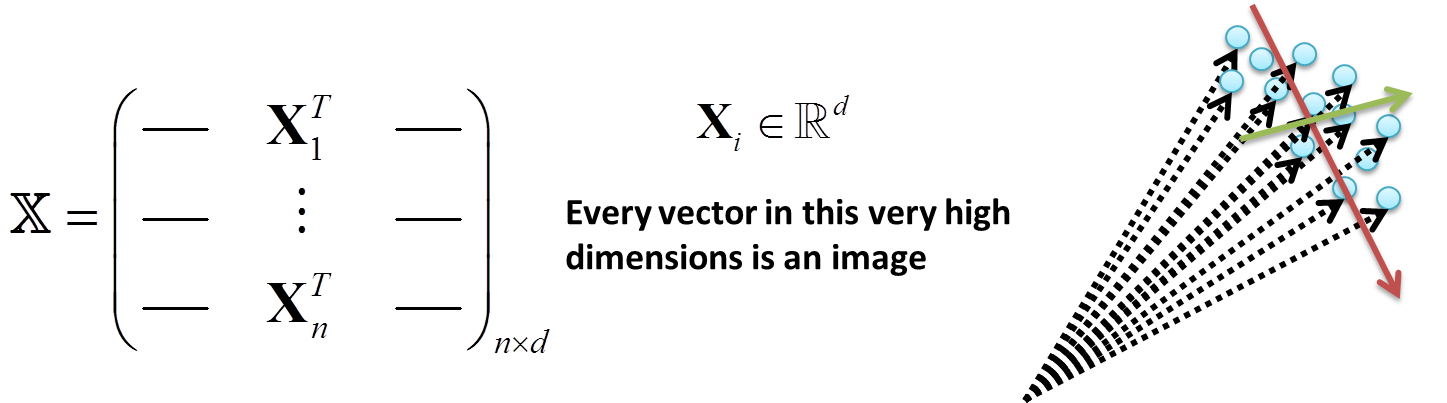
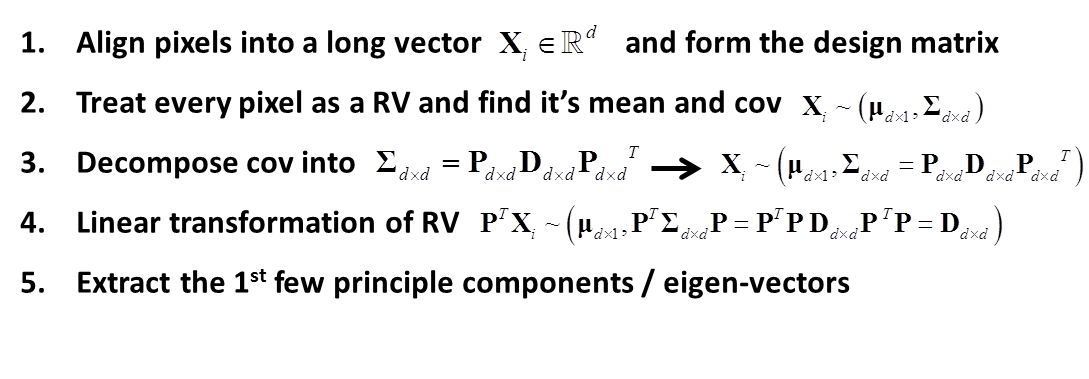
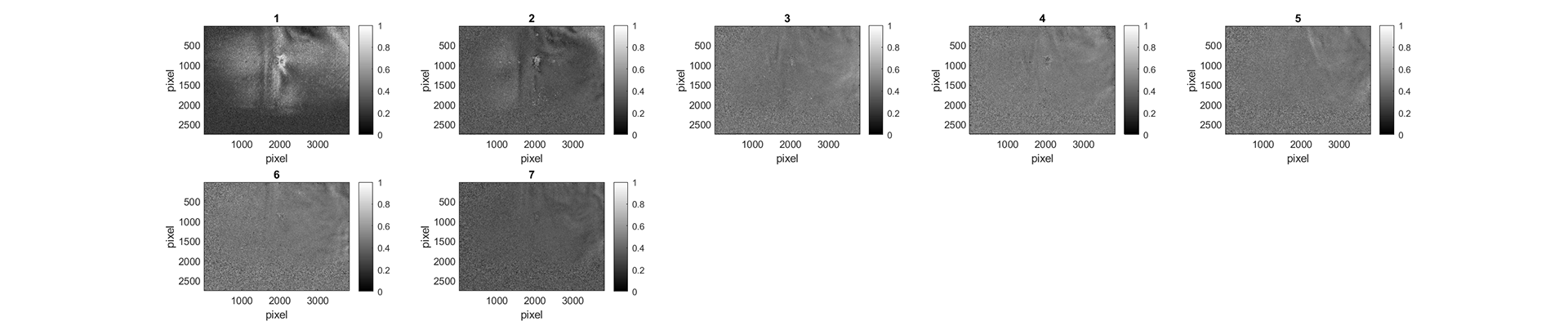
PCA - feature extraction by projecting data to eigen-vector directions
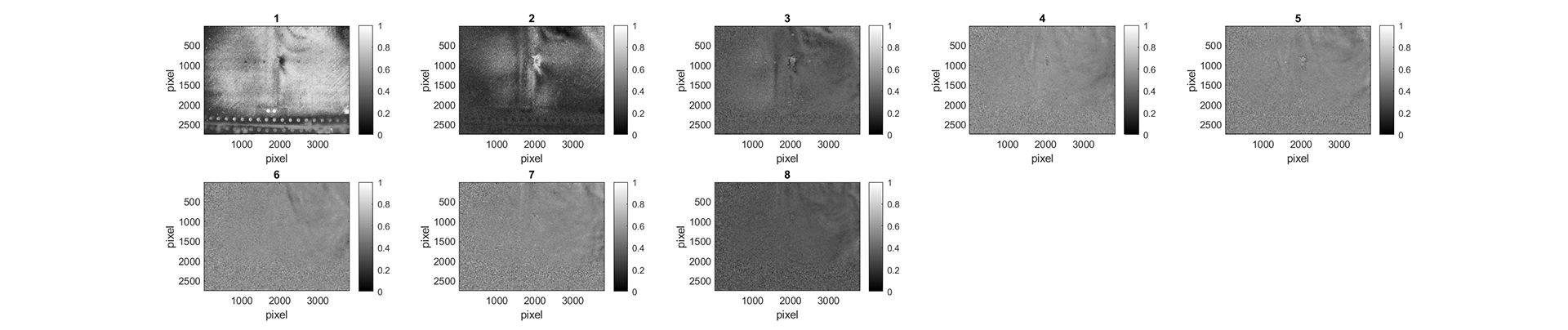
PCA - imaging fusion by superimposing data with eigen-value weightings